How Machine Learning is Revolutionizing Equipment Reliability
In today's industrial environment, unscheduled downtime can have disastrous consequences. A single breakdown can result in major delays, lost income, and, in certain situations, tarnished reputations in factories, production lines, and other industrial processes. In the past, maintenance techniques were reactive in nature, addressing issues as they surfaced. However, companies are currently moving toward predictive maintenance as a result of the introduction of cutting-edge technology like machine learning (ML). This creative method is changing the game by cutting downtime and elevating the dependability of the equipment.
What is Predictive Maintenance?
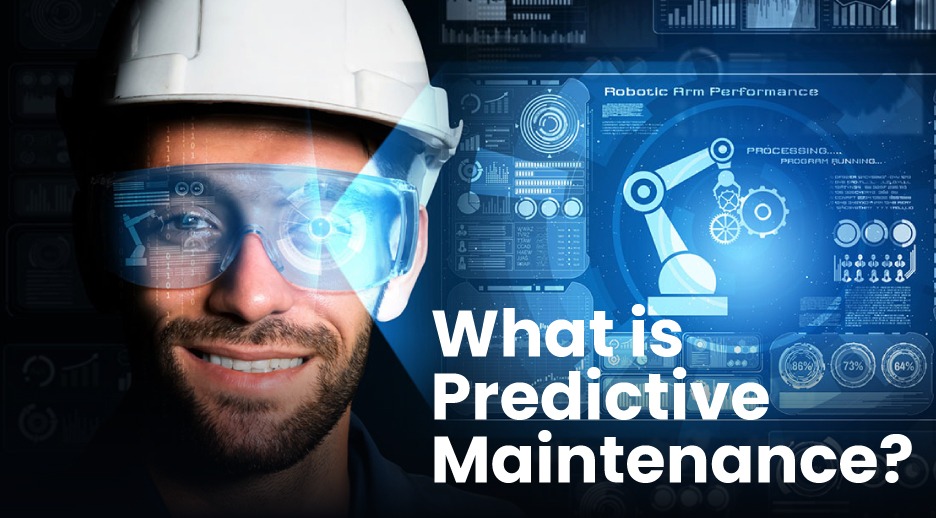
Using data analytic techniques to monitor machinery performance and condition in real time with the aim of anticipating when maintenance is needed, is known as predictive maintenance. In contrast to preventive maintenance, which plans maintenance at regular intervals, or reactive maintenance, which repairs after a problem occurs, predictive maintenance seeks to perform maintenance only when necessary. This reduces the possibility of unexpected equipment failure as well as needless downtime.
The data used for predictive maintenance comes from sensors that are integrated into equipment and monitor a variety of parameters, including vibration, pressure, and temperature. Machine learning algorithms can identify trends and abnormalities in this data that point to the possibility of a breakdown in the future. This enables businesses to resolve problems before they result in expensive failures
The Role of Machine Learning in Predictive Maintenance
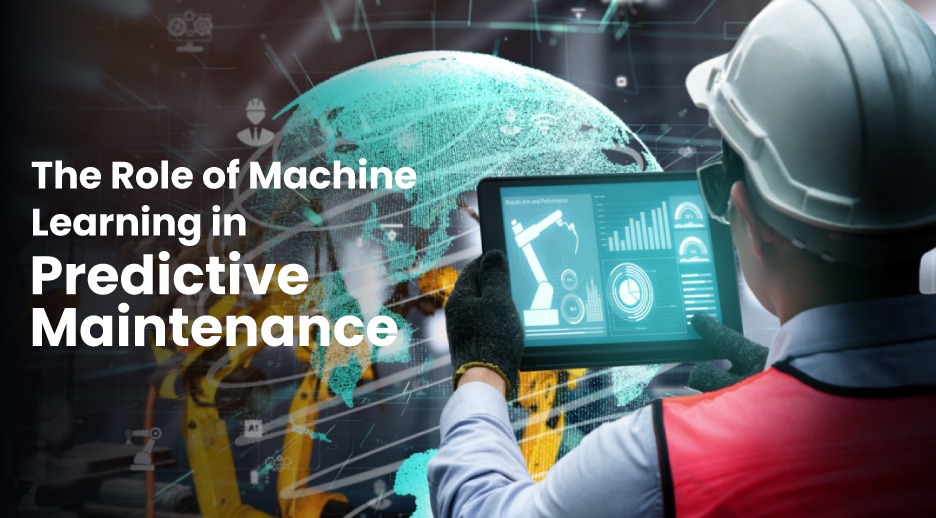
Machine learning is at the core of predictive maintenance systems. Its ability to process vast amounts of data quickly and identify trends and patterns makes it indispensable in modern industrial operations.
Here’s how machine learning enhances predictive maintenance:
- Data Collection and Analysis: Robots that have sensors installed continuously produce data about the tremors, heat, and pressure they experience. ML algorithms are capable of processing large amounts of data more quickly than traditional analysis methods because of their sheer bulk. They study patterns in both past and current data, looking for even the smallest discrepancies that can indicate a possible equipment failure.
- Anomaly Detection: The capacity of machine learning to identify anomalies is one of its most useful characteristics in predictive maintenance. Machine learning algorithms are able to recognize when a machine begins to function outside of its typical range by examining its historical behavior. A slight increase in vibration, for instance, could be a symptom of bearing wear, while a spike in temperature could be a sign of an imminent electrical failure. Companies can solve small problems before they become more serious by detecting these anomalies early on.
- Predictive Modeling: Machine learning models make predictions about the probability of equipment failure based on the gathered data. As more data is fed into these models over time, they become more accurate at predicting when a machine will require repair. The maintenance procedure is more accurate since the forecasts are based on dynamic, ever-evolving patterns in the data rather than just a static threshold.
- Failure Mode Identification: Machines can malfunction in a variety of ways, and there may be multiple underlying causes. Algorithms for machine learning can be used to classify failure modes and identify the precise causes of possible malfunctions. This enables maintenance personnel to handle the specific issue at hand rather than carrying out a broader, sometimes superfluous, maintenance routine.
How Predictive Maintenance Reduces Downtime
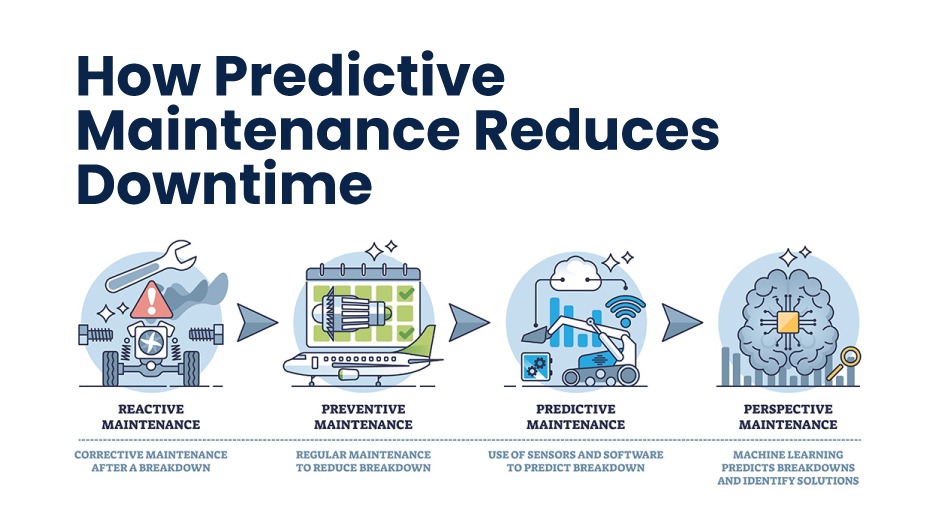
Unplanned downtime is one of the main problems that industrial operations encounter. Machine malfunctions can cause significant delays in deliveries, stop production lines, and cost large sums of money. Machine learning-powered predictive maintenance is essential in cutting down on this downtime.
Here’s how:
- Scheduled Maintenance Based on Actual Conditions: Because predictive maintenance is based on the machine's state rather than a preset schedule, it enables businesses to carry out repair only when it is truly necessary. By doing this, downtime and the expense of needless maintenance are both decreased.
- Early Detection and Prevention: Before they cause substantial harm, machine learning models identify the early indicators of failure. Businesses can avoid major malfunctions, which frequently need more time to remedy, by addressing minor problems early.
- Minimized Impact on Production: Companies can schedule maintenance during times of low output or downtime, assuring minimal disturbance to operations, since predictive maintenance gives advance notice of equipment breakdown.
- Improved Resource Allocation: Organizations can more efficiently deploy their resources if they know precisely which equipment need maintenance and when. By concentrating on the devices that actually require maintenance, technicians can cut down on time lost on pointless inspections or repairs.
The Financial and Operational Benefits of Predictive Maintenance
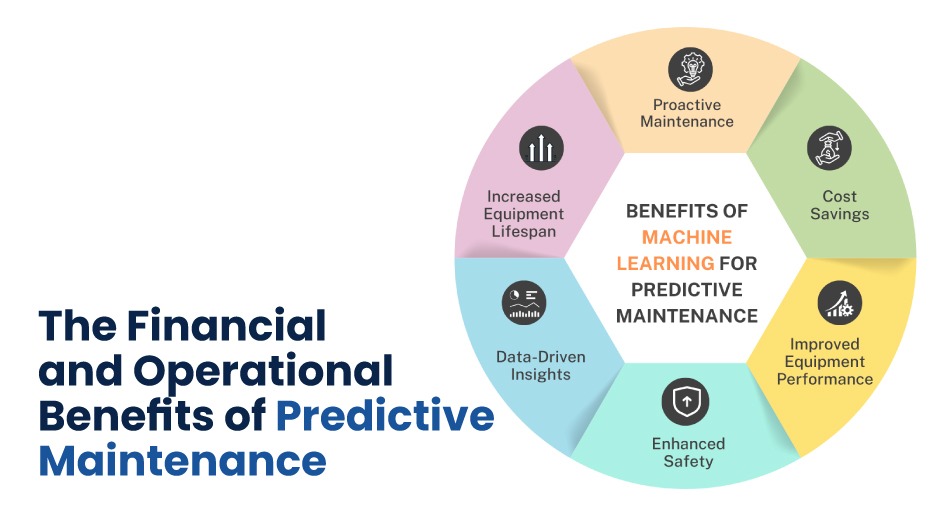
Predictive maintenance has significantly more financial benefits than only decreasing downtime. This method also helps to increase machinery longevity, lower maintenance costs, and increase operational efficiency. Here is a summary of some important advantages:
- Cost Savings: Total maintenance expenses can be lowered by up to 30% with predictive maintenance. Businesses can save money on repairs and prolong the life of their equipment by only doing maintenance when it's absolutely essential. Preventing catastrophic breakdowns that necessitate expensive emergency repairs is another benefit of early issue discovery.
- Extended Equipment Lifespan: Machine learning predictions used to guide routine maintenance can prolong the life of machines in optimal operating condition. Equipment is kept in better operating order and has a longer useable life when minor issues are prevented from getting worse.
- Improved Safety: Equipment malfunctions put workers' safety at risk in addition to causing downtime. Predictive maintenance contributes to a safer workplace by anticipating possible problems before they arise and lowering the risk of accidents brought on by broken equipment.
- Higher Productivity: Businesses can continue to operate at higher levels of productivity if there are fewer unplanned malfunctions. By assisting in the fulfillment of production schedules, predictive maintenance enhances customer happiness and enhances the reputation of the company.
- Data-Driven Decision-Making: Machine learning offers useful insights into the functionality of equipment. Businesses may make better judgments about their maintenance plans, production schedules, and upcoming capital expenditures for new machinery thanks to this data-driven strategy.
Challenges of Implementing Predictive Maintenance
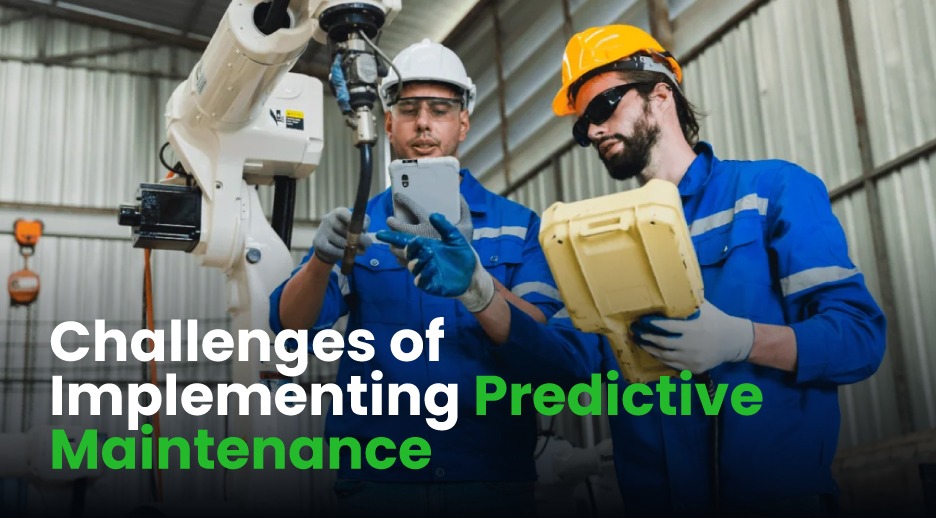
Predictive maintenance has several advantages, but putting this system into place might be difficult at times. A substantial investment in sensors, software, and data infrastructure is needed for the initial setup. Additionally, businesses want qualified workers with the ability to oversee and decipher data analytics and machine learning models.
Moreover, the quality and quantity of data gathered affects how accurate the predictions are. Inaccurate forecasts from poor data may cause premature maintenance or overlook problems. As a result, it's imperative that businesses make an investment in top-notch sensors and guarantee appropriate data collection procedures.
The Future of Predictive Maintenance
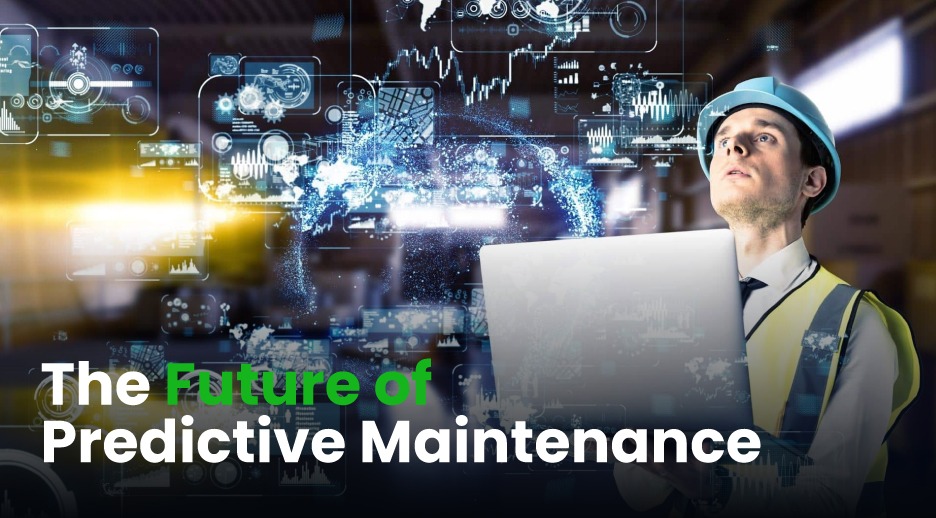
Predictive maintenance appears to have an even brighter future as machine learning technology advances. The capacity to monitor machines in real-time and produce even more accurate forecasts will be further enhanced by emerging technologies like edge computing, 5G, and the Internet of Things (IoT).
Additionally, machine learning models will advance with the collection and sharing of more data within businesses. Companies all throughout the world may benefit from predictive maintenance by maximizing equipment durability and reducing downtime if it were to become the industry standard.
Conclusion
Machine learning-powered predictive maintenance is transforming how industries handle equipment reliability. This technology is lowering costs, increasing operational efficiency, and decreasing downtime by enabling businesses to anticipate and avoid malfunctions before they happen. Predictive maintenance has long-term advantages that greatly exceed the initial expense required to adopt it. Predictive maintenance will become more and more important in the future to guarantee that industries can run smoothly and effectively while lowering the risks related to equipment breakdown.